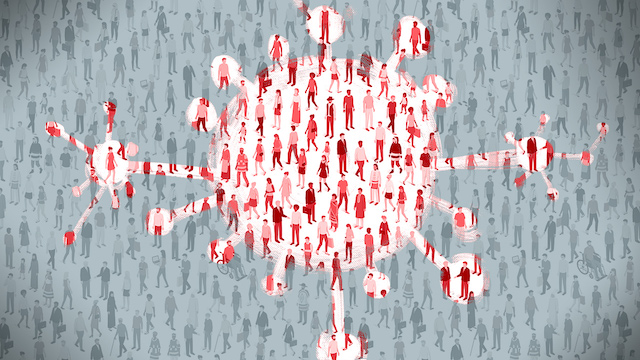
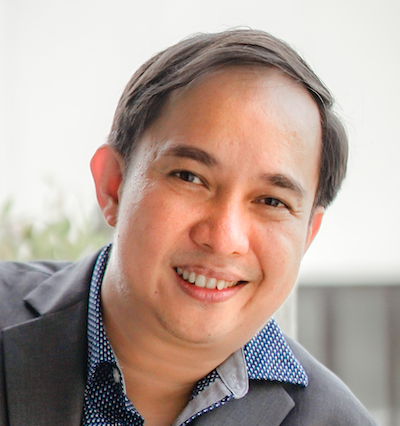
Knowing the incipient trajectory of the growth curve will give us hints on the potential of the epidemic; from the exponential curve, one can also infer the approximate reproduction number (R0) of a disease that measures its transmission potential. It has been established using empirical data, data-driven models, and equation-based models, among others, that during the initial infectious phase of an epidemic the growth of the number of infected individuals follows an exponential curve.
The initial growth, of course, is expected to peak after some time, and then flatten. Eventually, we will observe a logistic curve (or an S-curve). Thus, for policymakers keen to have a more objective and informed way to combat the spread of an epidemic, the following questions need to be answered:
- When will the exponential growth curve reach the peak?
- When will it start to flatten and approach the S-curve indicating that the number of infected individuals are not anymore increasing?
- Do our mitigating strategies such as the social distancing measures (e.g. ECQ or enhanced community quarantine) work?
One sure way we can answer these questions is if we can sufficiently collect and analyze the right set of data that will provide us with the true state of the nation in our fight against COVID-19. This means that we also need to collect accurate data. For this to happen, we need to deploy the right number of “sensors” (test kits) that will allow us to acquire the right number of samples that can represent our population.
Below, we explore various scenarios to drive this point home.
Where are we now? How many tests do we need to conduct? Why do we need to do it?
1. Where are we now? If the growth of the number of COVID-19 infected individuals is exponential (during this infectious period) then the number of individuals to be tested must be exponential, too.
The first figure shows that we can forecast this based on exponential growth estimates using a ratio and proportion (scaled exponentially) of the number of cases reported by the DOH and the number of tests administered (which is our current constraint).
Given the numbers for confirmed cases and tests administered in the last two days, we conjecture that the actual number of undetected infected cases *likely* have not deviated from the trend in previous days (as of Saturday, March 28, 2020). Why? On Saturday, we almost tripled the number of confirmed cases from the day before (272 confirmed cases from 96 confirmed cases). However, we also just tripled the number of individuals tested (399 tests from 140 tests), and yet the ratios between the confirmed cases and number of individuals tested are approximately the same: 272:399 ~ 96/:140 ~ 0.68.
If the inference above holds, then the number of COVID-19 confirmed cases that will be reported from March 29 to April 4 will continue to be dependent on how many tests will be administered in the same period. If it is going to be 400 individuals tested per day on Sunday, March 29, expect a report of confirmed cases within 1,259. Then by Tuesday, March 31, this could be 1,629.
If 1,000 tests per day were conducted starting March 29, then there would be 1,722 confirmed cases the day after, and by Tuesday, 2,648 such cases. If by some kind of miracle, we conduct 49,400 tests by March 31, then we can expect to have 23,000 infected cases to be reported by then.
2. How many tests do we need to conduct to show that the exponential growth still holds or that it is starting to peak/flatten approaching the expected logistic curve (S-curve)?
A lot. Our backlog as of March 28, is about 17,000 tests based on the conditions stated above. By March 31, we would need almost 50,000, and by April 3, 100,000!
If it is indeed true that we need about 2-3 test kits per individual suspected of COVID-19, then we need approximately 200,000-300,000 test kits by April 3 to have a better sense of the growth trends of COVID-19 infected individuals in the country. If the confirmed cases are way lower than 23,000, then good – we may just be starting to peak and we are probably flattening the curve. If the number is significantly higher than 23,000, then we are doubling faster than every 2.15 days.
3. Why do we need to conduct mass testing?
A. Why not? We need to understand that if we don’t, patients can die before they even learn they are COVID-19 positive; and we’d be stealing from them their chance to live. Currently, about 50% of COVID-19 casualties are, on average, 3 days dead before their status (+/-) gets confirmed. One case even got confirmation 10 days after the person passed away (ABS-CBN report).
The average deaths per case reported in the Philippines level off at 7% (see above figure). Seven deaths per 100 reported cases, not considering the undetected individuals. This is 50% higher than the global average of 4.6% (28,000/614,000).
B. We need to test more individuals because if we don’t, more people will be compromised and our quarantine strategy might not be as optimized. We need to at least have a sense of the portion of our population who are asymptomatic but highly infectious.
C. If we do not have a clue on the state of our country with respect to the potential and prevalence of this epidemic, the disease and its first- and second-degree effects could persist for a longer time, and we would be suffering more than necessary. At the very least, knowing the right estimates can inform our policymakers whether or not we can already safely lift the ECQs.
It is important for our country to balance at least these 3 things:
- the physical health of the Filipino people (especially our frontliners who we are truly grateful for)
- our mental health and well-being
- our economy – especially our micro, small, and medium enterprises
Having the right data and understanding of what the data is trying to tell us can help our leaders and policymakers strike this needed balance. – Rappler.com
*Christopher Monterola is one of the Aboitiz Chairs in Data Science at the Aboitiz School of Innovation, Technology and Entrepreneurship of the Asian Institute of Management.